Introduction
Improving a model’s accuracy is a tricky task, with an open question — where do you focus your resources?
Following the data-centric AI path, always start with data — improve the data quality where needed, focus the training cycles where the model suffers and you should be on the right path. But how do you identify these pain points?
In a previous blog, we saw how analysis of model accuracy could be conducted with modern tools to find the pain points, but there are cases where even this might not be enough, and further evaluation steps are required.
Saliency Maps
In some cases, a statistical analysis, such as one based on a confusion matrix or a PR-curve, is even more convincing when backed up by understanding the spatial support for the model’s choices. Alternatively, in case of inaccuracies, understanding the grounds on which they were made could lead to required modifications and improvements in the data, that would finally lead to an improved output.
Saliency Maps provide the insight into what influenced the model’s decision the most.
Essentially, these are heatmaps outlining which parts of the image influenced the model more, and which were less important. Once we understand that, we can decide what and how to change, in order to improve the model’s accuracy.
Viewing Saliency Maps
Akridata’s Data Explorer offers an interactive platform, where the accuracy metrics lead directly to the data, saving valuable time in analyzing model’s accuracy. Extending the analysis with Saliency Maps, users can see the confusion matrix, review the images in each cell, and view corresponding saliency maps, as seen with the example below:
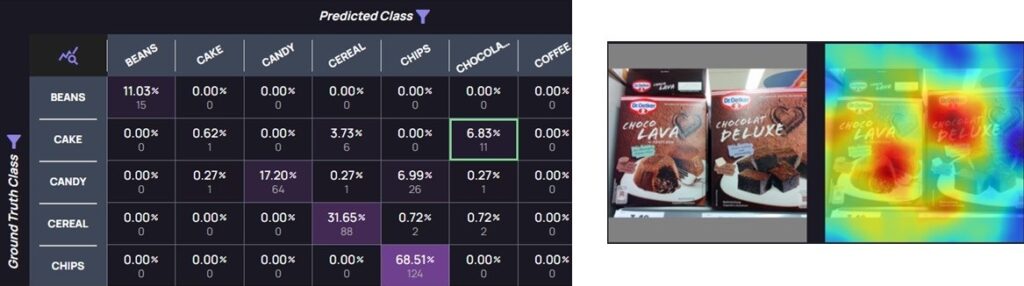
Left — a confusion matrix with a cell of mispredictions highlighted: Ground Truth=Cake, Predicted=Chocolate. Right — one of the images in the highlighted cell, with a Saliency Map, outlining why the misprediction occurred.
Based on what is seen in the Saliency Map, the user can decide how to improve the model’s accuracy, or if any change is needed at all.
Saliency Maps Use Cases
Below are two examples of classification tasks: a Profession classifier, and a Groceries classifier.
The initial Profession classifier had high accuracy scores, but Saliency Maps uncovered that model’s predictions weren’t based on the individual’s uniforms, as expected, but rather based on their head’s area. After balancing and enrichment of the training and test data, a new classifier was trained, which also showed high accuracy results, with decisions based on parts of the uniform, as illustrated below:
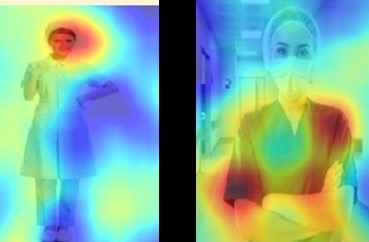
Profession classifier: (Left) Initial classifier — classification is based on heads’ area; (Right) Classifier after data enrichment — classification is based on uniform
Using Saliency Maps, it was evident that the initial Profession classifier needed further development, since a person’s head doesn’t hold relevant data, while the newer classifier could be used in production.
The initial Groceries classifier also showed high accuracy, and in the image below we can see the classification was based on the expected part of the image:
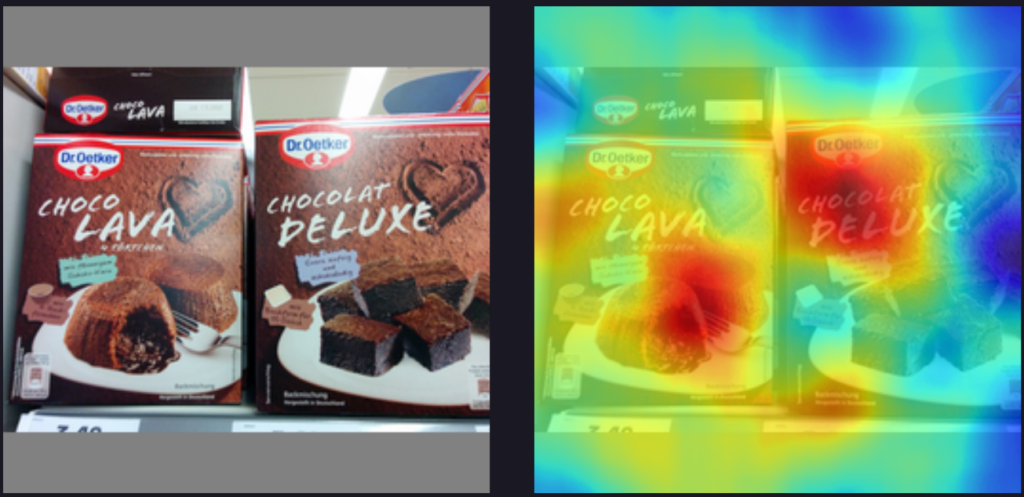
Grocery classification: Left — original image; Right — Saliency Map. Correct areas affecting the classifier
The above example reassured us that the decision was made based on the relevant part of the image, and the model was ready for production.
Summary
In this blog we saw how Data Explorer extends model accuracy analysis with Saliency Maps. This helps DS teams choose the best way on how to improve a model’s accuracy, or provide further assurance that the model’s predictions were based on the relevant parts of the image.
For a demo of Data Explorer, visit us at akridata.ai or click here to register for a free account.