Transform Manufacturing
Assembly Line Inspection
Many manufacturers have deployed vision systems into their manufacturing lines to automate quality inspection throughout the manufacturing process, but struggle to combat false positives and negatives.
Data Explorer uses deep learning to classify the images captured by your vision system to minimize the shipment of defective products while maximizing high yields – reducing the impact of defective product, increasing product quality and driving down operational costs.
WHO WE SUPPORT
Medical Devices
Agriculture
Automotive
Rail Transportation
Data Explorer seamlessly interfaces with your in-line inspection system, automating the generation of an autonomous, secondary set of decisions. This supplementary set of decisions serves as a benchmark for comparison with those made by your primary in-line system. With deep learning, you can analyze both the dataset and the model decisions to improve the models used by in-line systems.
- Pilot/NPI Manufacturing Lines:
Validate the performance and quality of the decisions made by your in-line system and provide data required for model explainability for Total Product Lifecycle Quality and regulatory requirements - Production Manufacturing Lines:
Have an independent second pass to minimize false positives/negatives to improve yields and provide continuous improvement to your in-line edge models through deep learning
HOW IT WORKS
Minimize False Positives &
Negatives with Data Explorer
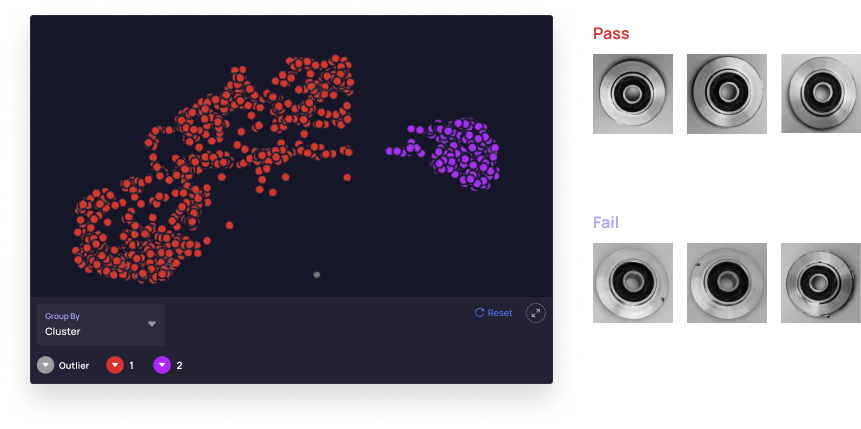
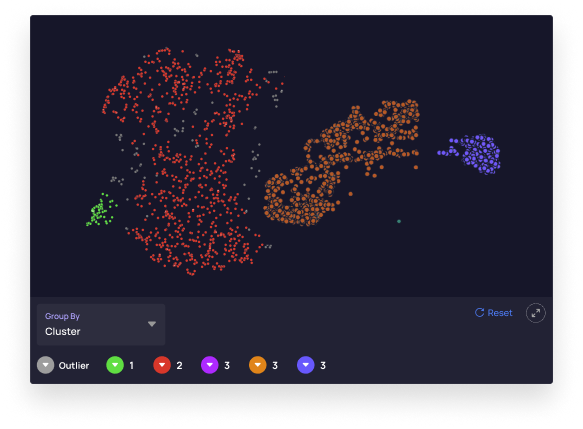
Overlaying Data Explorer’s findings with existing predictions enabled precise human intervention, refined inspection decisions, and reduced errors in part flagging.
CUSTOMER SPOTLIGHT
This Medical Device Company Decreased False Positives by 40%
A medical device company sought to improve accuracy and efficiency in its computer vision-based inspection lines. Existing computer vision technologies led to frequent false positives and negatives, impacting profits, increasing customer complaints, and inflating operational costs.
The company's in-line inspection system, efficient but inaccurate, fell short despite repeated model tuning. Real-world production conditions and a rigid, speed-focused approach caused incorrect part flagging, posing a challenge in balancing speed without sacrificing accuracy or risking defective product shipments.
This medical device company implemented Akridata to boost accuracy and efficiency in assembly line inspections.
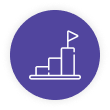
Leveraging Akridata’s Data Explorer, the pharmaceutical company achieved a notable 40% decrease in false positives and uncovered a 30% reduction in false negatives.
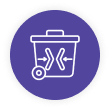
This improvement significantly reduced inventory wastage and prevented the shipment of defective products.
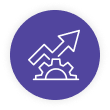
The implementation of Akridata’s solution elevated product quality standards, safeguarding the company’s esteemed brand reputation.
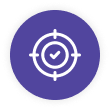
Improved operational accuracy efficiency and reduced recalls while cutting costs.