Heart disease continues to be the leading cause of death worldwide, making early detection and preventive measures essential. With the integration of machine learning (ML), healthcare professionals can now predict heart disease with unprecedented accuracy. This blog delves into the transformative impact of using ML for heart disease prediction, highlighting the benefits, key algorithms, and future implications.
Why Machine Learning Matters in Heart Disease Prediction
Machine learning algorithms have the ability to analyze complex datasets, uncover hidden patterns, and make predictions based on historical and real-time data. This is critical in healthcare, where early diagnosis can mean the difference between life and death. Traditional methods rely heavily on manual analysis, which can be time-consuming and prone to human error. On the other hand, machine learning provides:
- Enhanced accuracy: Through continuous learning from large datasets, ML models can refine their predictions, leading to higher accuracy in diagnosing heart disease.
- Efficiency: Machine learning reduces the workload for healthcare professionals by automating data analysis.
- Predictive insights: ML can anticipate potential health risks before symptoms manifest.
Key Machine Learning Techniques for Heart Disease Prediction
The application of machine learning in predicting heart disease typically involves a variety of algorithms. Below, we explore the most effective models:
- Logistic Regression: Commonly used for binary classification problems, this algorithm can predict the presence or absence of heart disease based on input features such as cholesterol levels, blood pressure, and age.
- Decision Trees: These algorithms break down decisions into branches, making it easier to understand how each factor contributes to the prediction.
- Random Forest: A more advanced ensemble method, Random Forest combines multiple decision trees to provide a more robust and accurate prediction.
- Support Vector Machines (SVM): Effective for classifying complex data, SVMs can create a decision boundary that separates classes (e.g., heart disease-positive and negative cases) based on various health metrics.
- Neural Networks: Particularly deep learning models have shown immense potential due to their ability to learn intricate patterns from massive datasets, including medical imaging and electronic health records.
Real-World Applications
The application of machine learning in heart disease prediction is already proving successful in various settings:
- Predictive Analytics Platforms: Hospitals and healthcare providers use ML-powered platforms to analyze patient data and forecast potential heart complications.
- Wearable Devices: Smartwatches and fitness trackers equipped with sensors can collect data on heart rate and activity levels, which ML models analyze to detect anomalies.
- Remote Monitoring Solutions: ML algorithms help in processing data from remote monitoring tools to alert patients and doctors about potential cardiac events.
Data Requirements for Effective Prediction
The success of heart disease prediction using machine learning depends on the quality and quantity of data:
- Patient Demographics: Information such as age, gender, and ethnicity can impact heart disease risk.
- Medical History: Past records of illnesses, surgeries, and genetic predispositions are crucial for training ML models.
- Lifestyle Factors: Data related to diet, smoking, and exercise habits contribute significantly to prediction accuracy.
- Medical Test Results: Metrics like blood pressure, cholesterol levels, and electrocardiogram (ECG) data play a pivotal role.
Ensuring data quality involves cleaning, pre-processing, and selecting relevant features. Proper data normalization and handling of missing values also improve the model’s performance.
Challenges in Using Machine Learning for Heart Disease Prediction
While machine learning holds promise, it is not without its challenges:
- Data Privacy and Security: Patient data must be safeguarded against breaches, which involves compliance with regulations such as HIPAA.
- Interpretability of Models: Some complex models, like deep learning neural networks, can be seen as “black boxes,” making it difficult for medical professionals to understand the reasoning behind predictions.
- Data Imbalance: Often, datasets may have a skewed distribution (e.g., more non-cases than cases of heart disease), which requires techniques like oversampling or the use of synthetic data to balance the training set.
Future Trends in Machine Learning for Cardiology
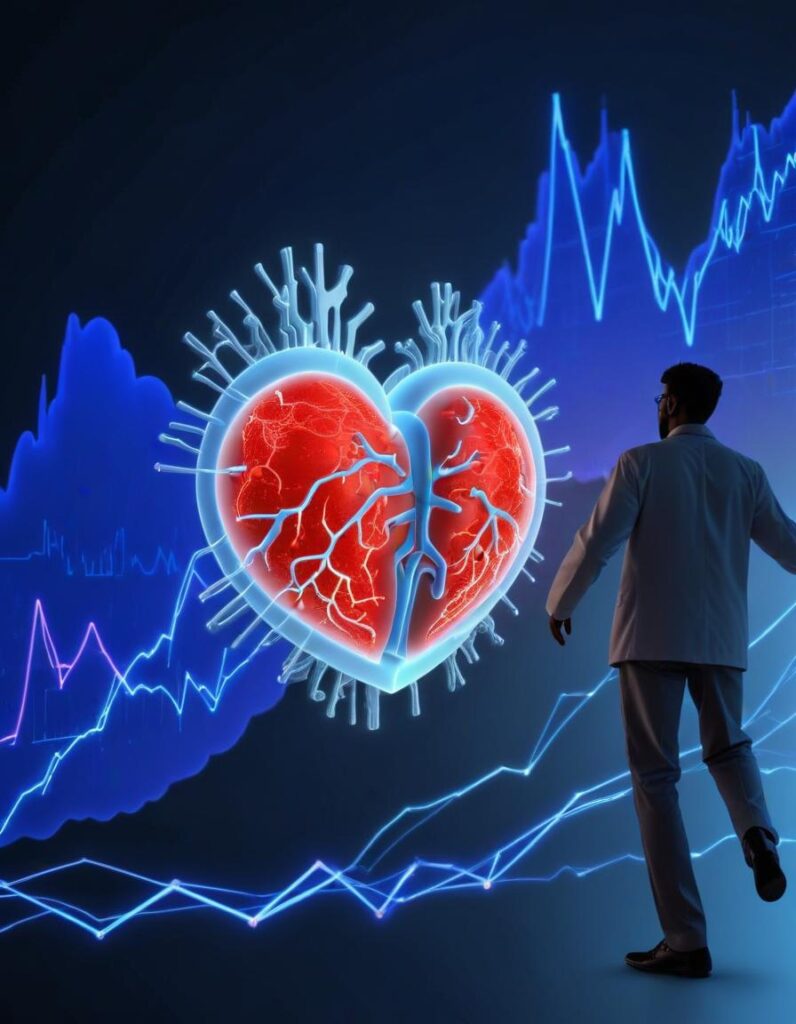
The field of machine learning for heart disease prediction is continually evolving. Here are some trends to watch:
- Integration with Genomics: ML models are starting to incorporate genetic data, offering a more personalized prediction of heart disease risk.
- Federated Learning: This approach allows models to learn from decentralized data sources without sharing patient data directly, maintaining privacy while improving model training.
- Explainable AI (XAI): With more focus on model interpretability, XAI techniques are being developed to make ML predictions clearer for clinicians.
- Enhanced Real-Time Monitoring: As wearable technology advances, ML models will process more real-time data, enabling proactive health interventions.
Case Studies: Success Stories in Predicting Heart Disease
Case Study 1: Cleveland Heart Disease Dataset Using data from the Cleveland heart disease dataset, researchers have successfully trained models to predict heart disease with high accuracy. Algorithms like Random Forest and logistic regression have been pivotal in achieving above 85% prediction rates.
Case Study 2: Google Health’s Deep Learning Model Google Health has developed deep learning algorithms that analyze retinal images to assess heart disease risk, demonstrating the power of AI in non-invasive medical diagnostics.
Conclusion: The Lifesaving Potential of Machine Learning
The potential of machine learning in heart disease prediction cannot be overstated. By enabling early diagnosis, more personalized treatment plans, and enhanced monitoring, ML is poised to reshape cardiology and healthcare at large. While challenges exist, ongoing advancements in data processing, algorithm transparency, and wearable technology integration promise to make heart disease prediction more accessible and effective. Embracing these innovations is a crucial step toward reducing heart disease mortality rates and improving global health outcomes.
No Responses