Reviewing the Current Data Set
Let’s take a look at the dataset we have.
For the purpose of the illustration, we will refer to the nuScenes dataset.
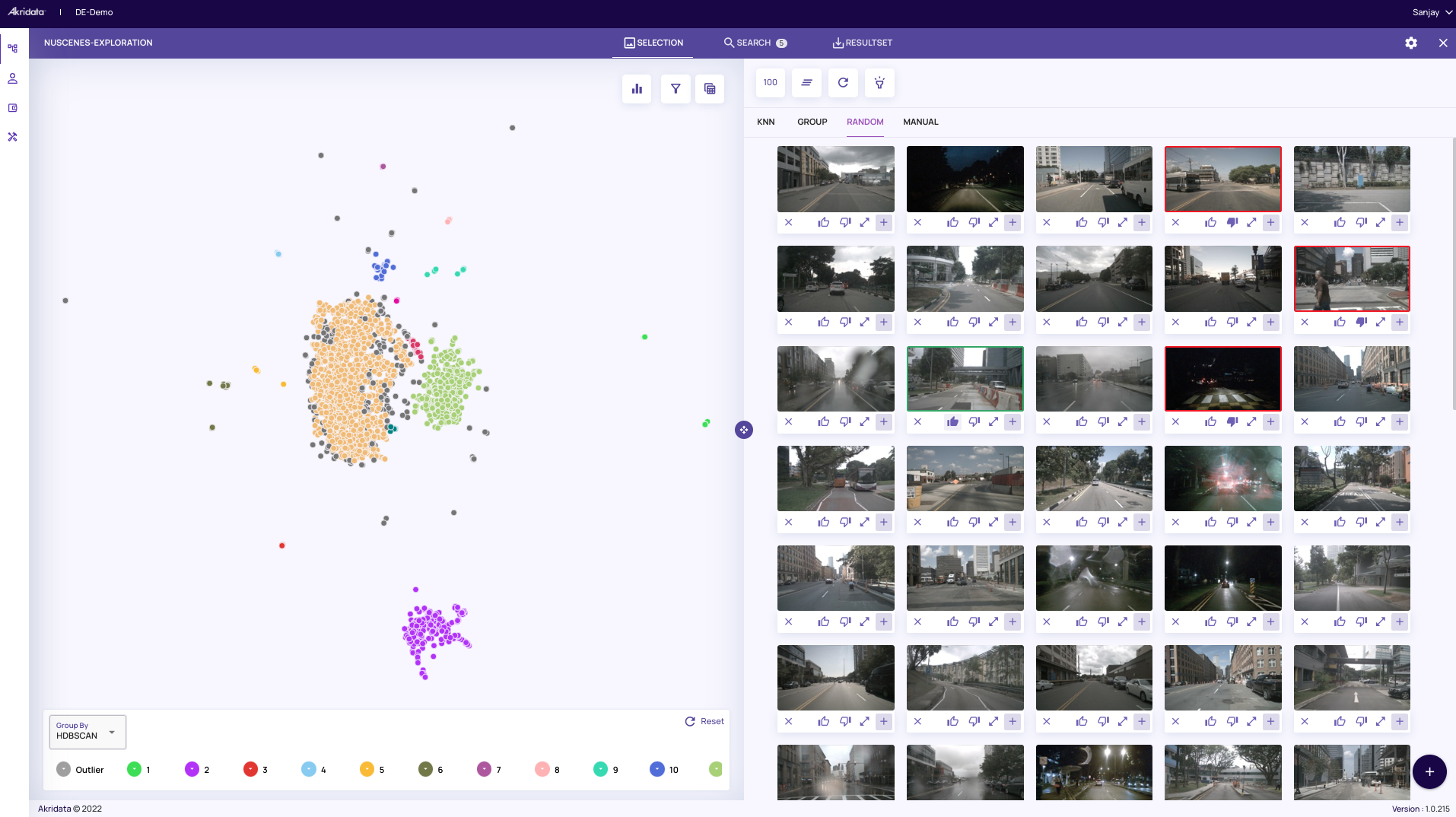
The images on the right are a random sample of the dataset which reflects different scenes – sunny days, traffic lights, pedestrian crossings, night shots, and rainy days.
Using Our Patch Search Feature
Let’s suppose you want to use our Patch Search feature to find images that have a traffic light.
You will see that the search results have faithfully captured the neighboring frames in the video sequence that have the traffic light.
As expected, these images are the nearest neighbors in the highlighted cluster map as shown below (by using our flashlight feature)
However, you want to give the labelers a dataset that has a diverse representation of the traffic light, not just multiple frames captured at one street corner.
Applying Coreset Sampling
To capture the diversity of scenes that have the traffic light, we can apply Coreset sampling and reduce the dataset in the feature space.
As shown below, we select Coreset in the sampling and the sampling fraction to 0.01 (1%) in the Tunables panel.
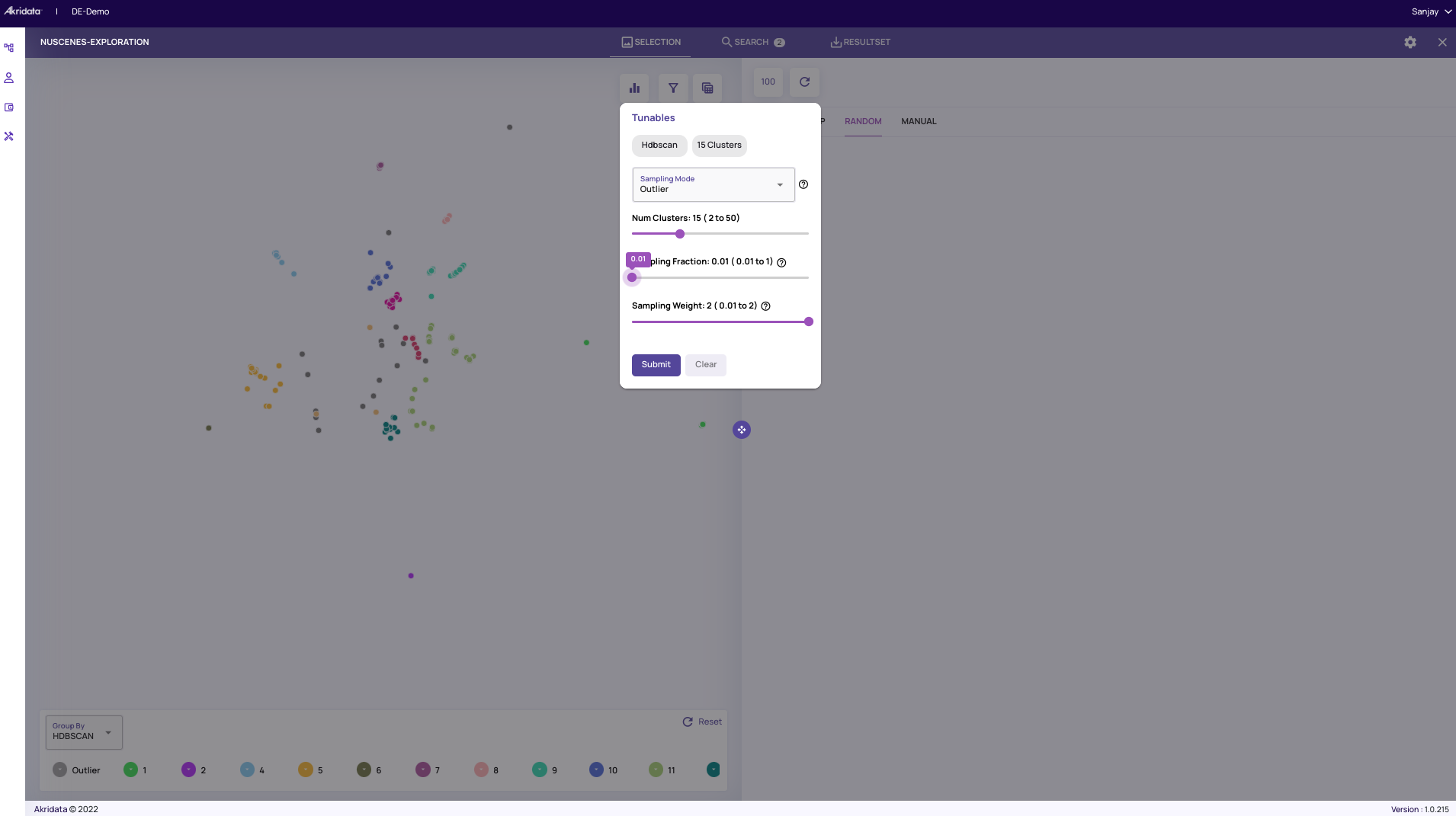
Finding The Traffic Lights
Now we run the same patch search, except that this time we are going to run it on the coreset sampled dataset by selecting “Apply Tunables”.
The search results now reflect images that have traffic lights from various scenarios and angles.
The search results shown are represented by the highlighted points (using our flashlight feature) in the overall cluster representation of the original dataset (100%).
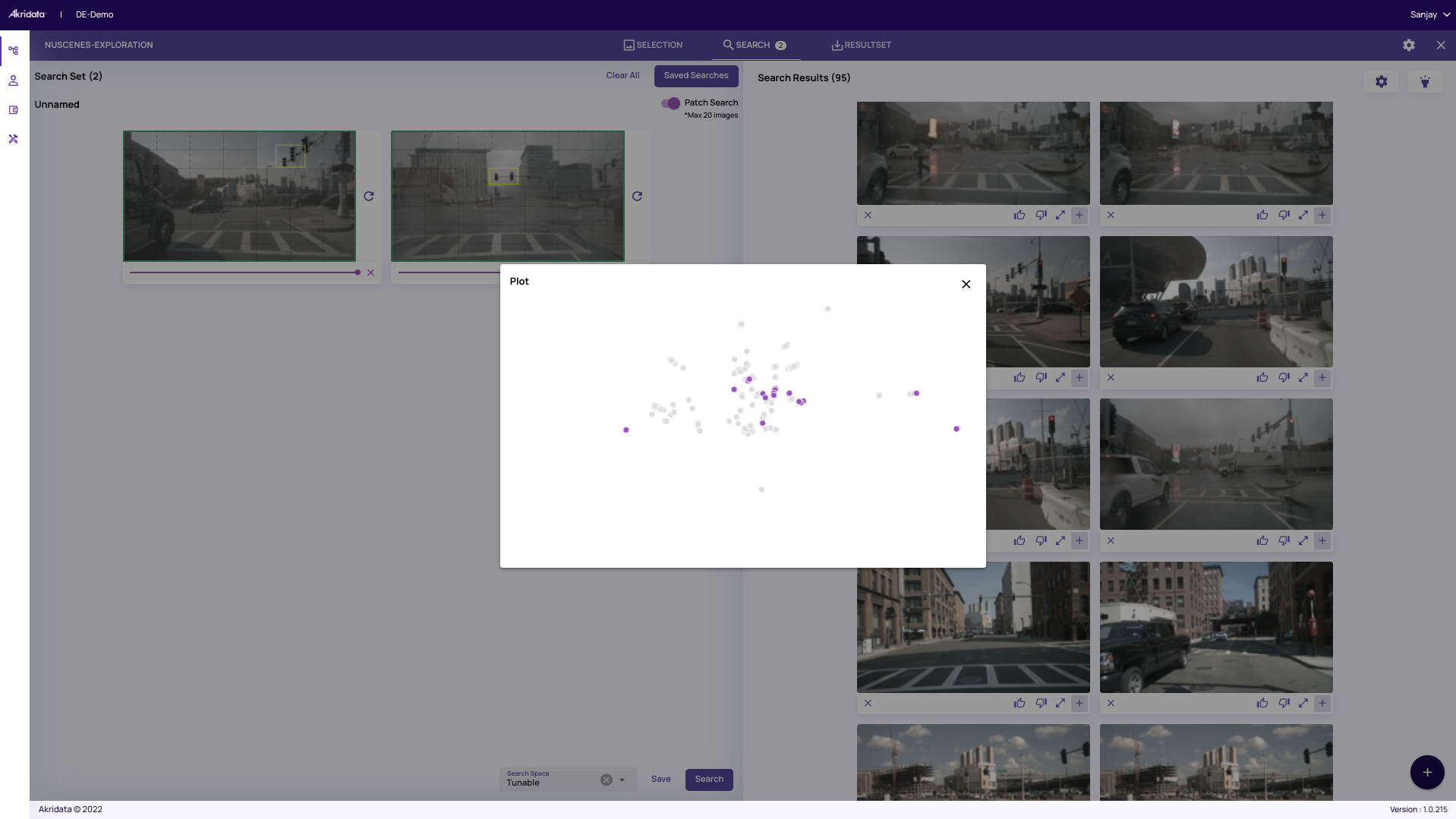