AI Computer Vision for Railways
Prevent Train Derailments with Akridata for Railways
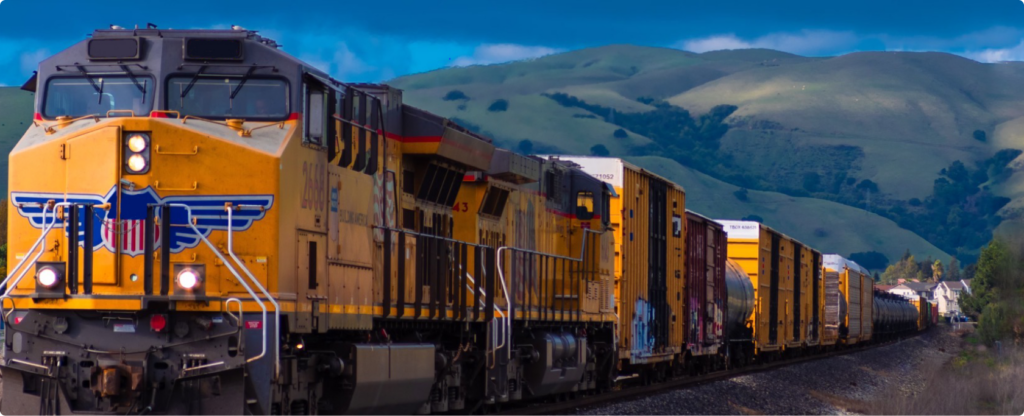
There are more than 1,000 train derailments per year in the United States, resulting in a significant loss of time, money, and — worst of all — lives.
SOLUTION
Automated Wheel Defect Detection
Problem
Railway companies gather millions of wheel inspection waypoint images. Yet, the shortage of sample data examples hinders effective wheel defect identification, compromising safety and efficiency.
Goal
Prevent derailments by enhancing models with synthetic images to improve the accuracy of defect detection.
Solution
Leverage Akridata to generate hundreds to thousands of synthetic images, enriching your dataset and enhancing your model's accuracy.
See how Akridata can help.
Integrated Railway Inspection System
Edge Collection & Inference
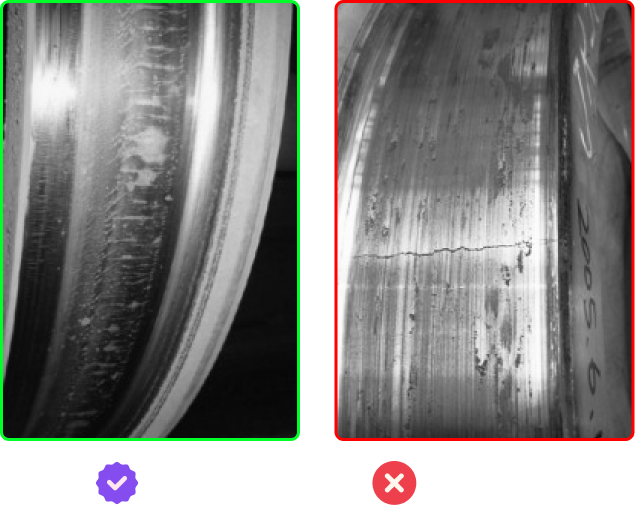
Edge Collection & Inference
Advanced Wheel Inspection
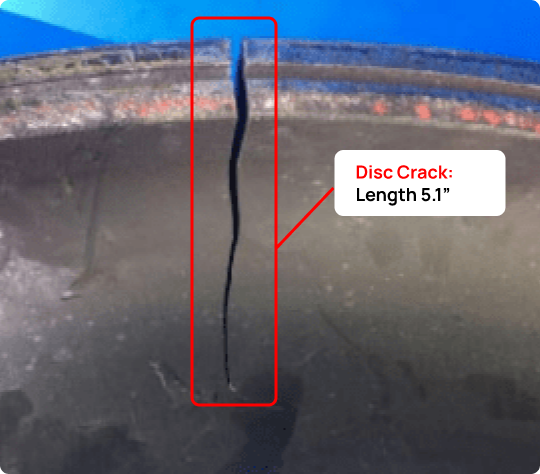
Advanced Wheel Inspection
Synthesizing Crack Variations for Enhanced Model Reliability
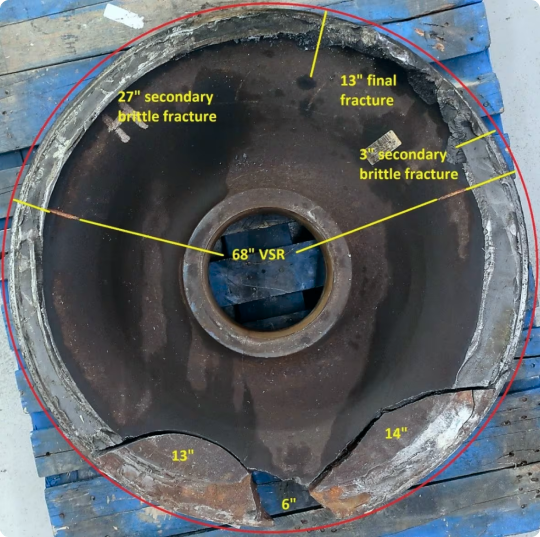
Synthesizing Crack Variations for Enhanced Model Reliability
Continuous Deployment and Monitoring
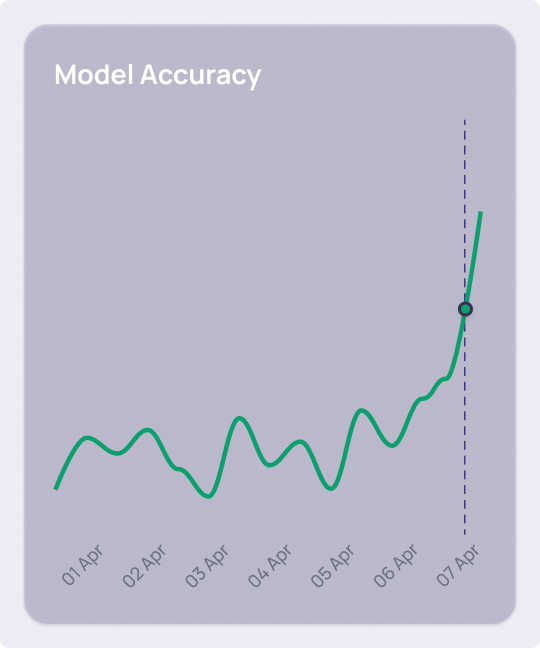
Continuous Deployment and Monitoring
Need More?
Akridata Visual Data Platform
Don’t have an in-house Data Science team for railway defect detection?
Akridata Edge provides ready-to-use railway models, rigorously tested and refined with millions of train wheel images, ensuring accurate defect detection.
Largest freight railroad company achieves 98.5% Model Accuracy
By using Akridata Data Explorer to refine and enhance their computer vision model, the largest freight railroad company increased the accuracy of their wheel inspection model to 98.5%. Additionally, since using Akridata, they have not experienced any instances of real-world wheel cracks that went undetected by the model.
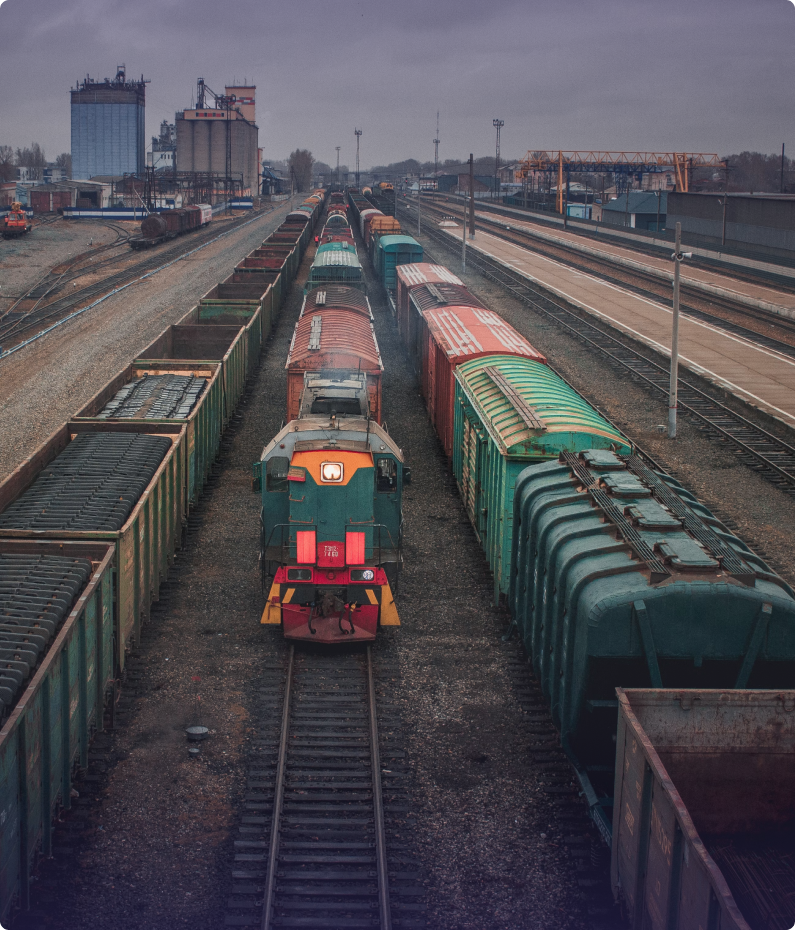